What caught our attention at MIT’s festival of sports data
Once dubbed “Dorkapalooza” by ESPN's Bill Simmons due to the focus on data in sports, the MIT Sloan Sports Analytics Conference has become a fixture in the calendar for those interested in the evolving intersection of technology and sports.
I find it stands out from other sports conferences, thanks to its strange but endearing mix of research papers (with my reactions ranging from “that’s bonkers” to “ooh, that’s interesting”), presentations from practitioners (my favourite sessions), and keynotes from industry “names”. It’s also one of the few conferences that seems to easily mix the athletic performance side of the industry with the commercial side. As a result, it really made me think about how more can be done to use performance data in a commercial environment. For example, how broadcasters can bring the latest thinking in match analysis into their coverage, or how players might use their performance stats commercially.
These were my main takeaways.
Blending datasets can reveal new opportunities
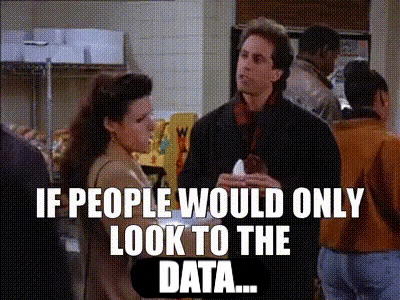
The idea of enriching data – the process of taking first party data and then combining it with external sources – is not new. For example, this has often been used to show potential sponsors the demographic breakdown of a team’s fanbase. However, this has normally been done by combining relatively few data sets. For example, the ZIP code or postcode data of ticket buyers and socio-economic data from vendors, such as Experian.
One of my favourite presentations at the event was from Tiffany Krause, senior manager of fan data science and analytics at NFL. She explained how the league is building a lead-scoring model to help teams identify the local businesses that are most likely to be converted into sponsors. By cross-referencing a large number of datasets – including business location, company performance, ticketing data and employment records – teams can rank potential sales leads based on travel time to the stadium, revenue growth, numbers of fan employees and even fan employees in decision-making positions.
I really liked the creativity of the approach and the strategy that had gone into which data sets to use, as it showed a real clarity of thought around how the data was going to be applied to a real-life business challenge. It’s also notable as an example of how AI can be leveraged in a real-world scenario to provide insights that might not otherwise be realised due to the volumes of data involved.
Tech will bring pro tier broadcast analytics to more games
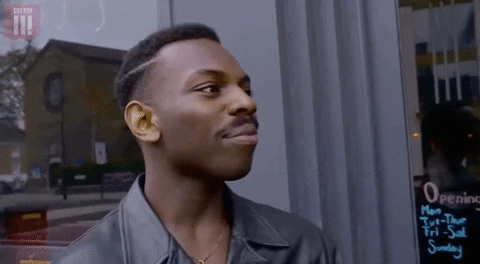
With an ever-increasing number of lower league matches being televised, thanks to partnerships like Sky Sports' recent deal with the EFL (which promises over 1,000 games to be broadcast per season), fans now expect the same level of in-depth analysis seen in top-tier matches to be applied across all games.
However, there’s a problem: it’s expensive to have high-quality data-gathering capabilities installed across lots of different venues. A recurring theme at the event was that broadcast feeds can provide the solution.
The trouble with using tracking data obtained from broadcast footage has been that it's inherently incomplete – thanks to players being outside the broadcast camera's frame or close-ups focusing on individuals. Instead, Harry Hughes from Stats Perform presented a research paper at the event that showed how generative AI could be used to predict the locations of players, even when out of shot on the broadcast feed. The idea is to create a more complete dataset and allow analysts to assess all of the on-pitch action – even if it wasn’t captured on the broadcast feed.
This feels like it could solve a genuine problem. But I’m keen to see what the variance is between the data that is created by generative AI and the actual recorded data. The point at which the variance becomes small enough to overlook, considering the cost savings, will be pivotal for its adoption.
What if it was easier to share data?
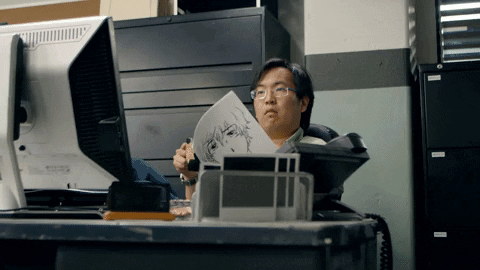
One of the things that’s held sports rightsholders back from making the most of their data is the challenge of data sharing. This issue often arises from privacy concerns and understandable hesitations regarding who should have access to and work with the data, as well as technical limitations.
Michelene Rabbitt, sports and music industry principal at Snowflake, talked about data sharing falling into two key categories.
Firstly, by discussing the ‘who’, which means setting the right permissions and framework at a very granular, even role-based level. This will allow the right people to have access to the right data at the right time. She gave the example of business leaders having access to certain data alongside technology teams – which means that the analytics tools need to be both easy to use for non-technical staff, and accessible from a cost perspective.
Secondly, she discussed the ‘what’, with regards to the type of data being shared. Perhaps that’s raw data that advanced users can manipulate themselves. Or maybe, if data is being shared with non-technical staff, a more consultative and storytelling approach is needed to help the recipients understand how to use and interpret the data.
This made me think of the different use cases that could be facilitated if data sharing was made much simpler both within and between organisations. For example, data clean rooms could be used to combine anonymised fan data from a team with customer data from a sponsor, in order to see how much overlap there is between the two – effectively establishing ‘sponsor fit’. Alternatively, a team might share an athlete’s individual performance data with them, enabling that athlete to use that data on social media as part of an endorsement deal.
New skill sets are needed in sports organisations
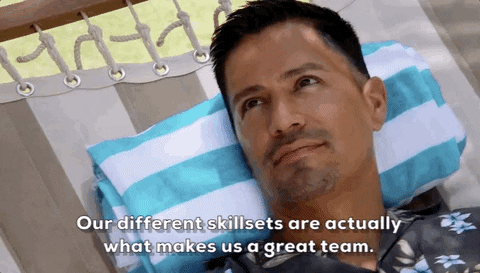
At an event organised by a university, it was no surprise that there was lots of discussion about the skill sets that would be needed within sports organisations in the near future. However, it was also clear that though some sports organisations (especially in the US) have invested heavily in people with data science backgrounds, many teams and leagues are still lacking the teams and skill sets to take advantage of the increasing amount of data that’s being produced.
For me, there were three takeaways about skills from the event. The first was the importance of curiosity when working with data. Dejan Mitkovski, VP of data analytics at FanDuel, identified curiosity as a critical trait that he looks for in hires. This means finding people with the ability to look at things differently and ask: “what if we did x?” as well as ensuring that the organisation fosters a culture that allows such curiosity to flourish.
The second is the growing need for ‘product owners’ within teams and leagues – people who can manage the relationships with vendors, and the technology they provide. With this capability increasingly being done internally (rather than through an agency), we see it as an important position, as sports organisations aren’t generally set up to build technology themselves (and neither should they be). Instead, they need people who know how to effectively work with large tech companies, and can also get the best out of smaller startups that they work with.
Finally, people often focus on the value of prompt-writing when working with AI, but I think we’re going to see increasing value put on people who know how to frame and explain recommendations from an AI model when making a decision. Whether people perceive the output from an AI model as credible is going to be increasingly important. So the skills required to ‘sell’ the model’s recommendation, but also understand its limitations, will therefore become more valuable. For example: “This model has worked well in previous similar situations. This scenario is slightly different because of x and y, but we are still confident that the model can be applied.”
Looking ahead
For more insights, including on how AI can be applied in a commercial sports setting, join us at this year’s Sports Loft Showcase on May 8, 2024. If you're a rightsholder or investor and would like to join us at the event, contact me about attending via charlie@sportsloft.co.
"